Lies, damn lies, and marketing data: 4 ways survey results are skewed
A marketing executive wonders why we seem to implicitly trust studies, even though their numbers can be so easily manipulated.
Four out of five dentists recommend sugarless gum for their patients who chew gum? Prove it. Show me the data—all the data.
It’s not that numbers lie; people do—or they mislead, at least, consciously or not. People are often wrong, inexperienced, shortsighted, and biased. People bring unspoken agendas to the table, consciously or unconsciously—a way of looking at numbers whereby they are hoping to be proved right.
We’re all guilty of it, and yet, most people trust data. Every day, I see people mindlessly sharing, tweeting, and retweeting survey and poll data, Web analytics, and infographics as if the Almighty had handed them down like the 10 Commandments.
Some are very authoritative; others are not. And that has me wondering whether we (as a profession) bring enough skepticism into the workplace.
I thought we should take a look at just a few of the many ways data can be skewed. Perhaps you’ll agree with some of these and add a dash of skepticism to your daily routine. Better yet, perhaps you can add to this list.
Become a Ragan Insider member to read this article and all other archived content.
Sign up today
Already a member? Log in here.
Learn more about Ragan Insider.
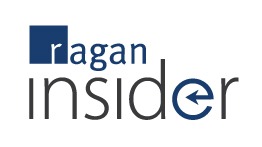