How machine learning can perfect your pitching
Here’s how to use technology—much of it free and user-friendly—to elevate your media relations and improve your journalist outreach.
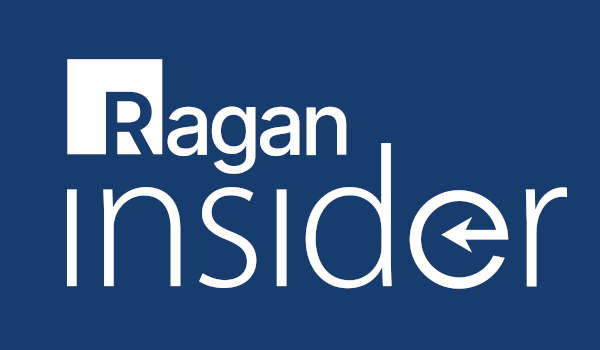
You may be dazzled, spooked or annoyed by the ability of online retailers to predict which products you’re interested in purchasing—but what if you could play the same game?
Whether it’s Amazon recommending soap or Netflix suggesting a movie, more companies are making personalized predictions using variations on a machine learning tactic called market basket analysis. This technique uses an algorithm that sorts through behavioral data to determine how frequently certain actions (purchases, views, etc.) are associated with other actions. The algorithm provides the statistical likelihood that if one action takes place, another desired action is likely to follow.
Sophisticated algorithms aren’t limited to shopping carts or movie recommendations, however. The same principles can be applied to all kinds of behaviors and disciplines—including PR. PR agencies can use algorithms, AI and machine learning to better target journalists by predicting which ones are likely to write about specific topics based on what they’ve previously written. This might sound expensive or beyond your expertise, but it’s neither. You can harness the power of machine learning with free software and data that you probably already have.
Become a Ragan Insider member to read this article and all other archived content.
Sign up today
Already a member? Log in here.
Learn more about Ragan Insider.
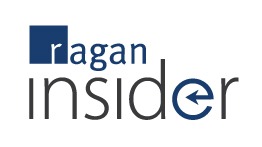
Tags: AI pitching, pitching, pitching tips, PR, PR pitch, PR technology, public relations